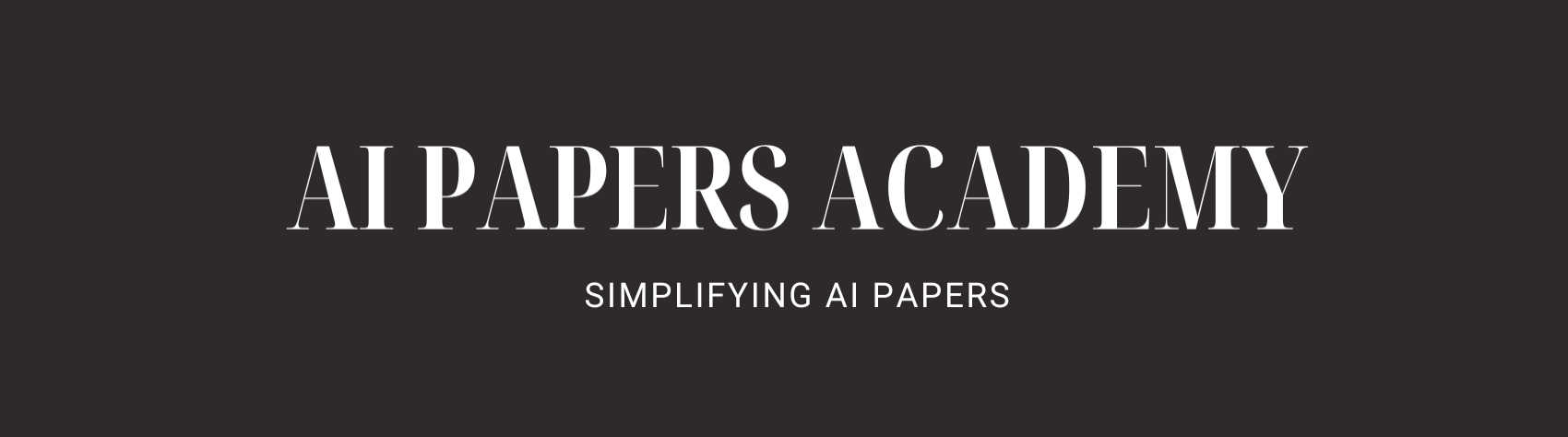
Looking for a specific paper or subject?
Recent Posts
- Sapiens: Foundation for Human Vision ModelsIntroduction In this post, we dive into a new release by Meta AI, presented in a research paper titled Sapiens: Foundation for Human Vision Models, which presents a family of models that target four fundamental human-centric tasks, which we see in the demo above. Fundamental Human-centric Tasks In the above figure from the paper, we can learn about the tasks targeted by Sapiens. Impressively, Meta AI achieves significant improvement comparing to prior state-of-the-art results for all of these tasks, and in the rest of the post we explain how these models were created. Humans-300M: Curating a Human Images Dataset The… Read more: Sapiens: Foundation for Human Vision Models
- Mixture of Nested Experts: Adaptive Processing of Visual TokensMotivation In recent years, we use AI for more and more use cases, interacting with models that provide us with remarkable outputs. As we move forward, the models we use are getting larger and larger, and so, an important research domain is to improve the efficiency of using and training AI models. Standard MoE Is Enough? A method which we already touched in a previous post, that became popular for large language models (LLMs), but later on also for computer vision, is Mixture-of-Experts (MoE), which helps to increase models size, without a proportional increase in computational cost. However, it comes… Read more: Mixture of Nested Experts: Adaptive Processing of Visual Tokens
- Introduction to Mixture-of-Experts (MoE)In recent years, large language models are in charge of remarkable advances in AI, with models such as GPT-3 and 4 which are closed source and with open-source models such as LLaMA 2 and 3, and many more. However, as we moved forward, these models got larger and larger and it became important to find ways to improve their efficiency. Mixture-of-Experts (MoE) to the rescue. Mixture-of-Experts (MoE) High-Level Idea One known method that has been adapted with impressive success is called Mixture-of-Experts, or MoE in short, which allows to increase models capacity without a proportional increase in computational cost. The… Read more: Introduction to Mixture-of-Experts (MoE)
- Mixture-of-Agents Enhances Large Language Model CapabilitiesMotivation In recent years we witness remarkable advancements in AI and specifically in natural language understanding, which are driven by large language models. Today, there are various different LLMs out there such as GPT-4, Llama 3, Qwen, Mixtral and many more. In this post we review a recent paper, titled: “Mixture-of-Agents Enhances Large Language Model Capabilities”, which presents a new method, called Mixture-of-Agents, where LLMs can collaborate together as a team, and harness the collective expertise of different LLMs. So, instead of using a single LLM to get a response, we can get a response that is powered by multiple… Read more: Mixture-of-Agents Enhances Large Language Model Capabilities
Top Posts
-
What is YOLO-NAS and How it Was Created
In this post we dive into YOLO-NAS, an improved version in the YOLO models family for object detection which was precented earlier this year by Deci. YOLO models have been around for a while now, presented in 2015 with the paper You Only Look Once, which is what the shortcut YOLO stands for, and over…